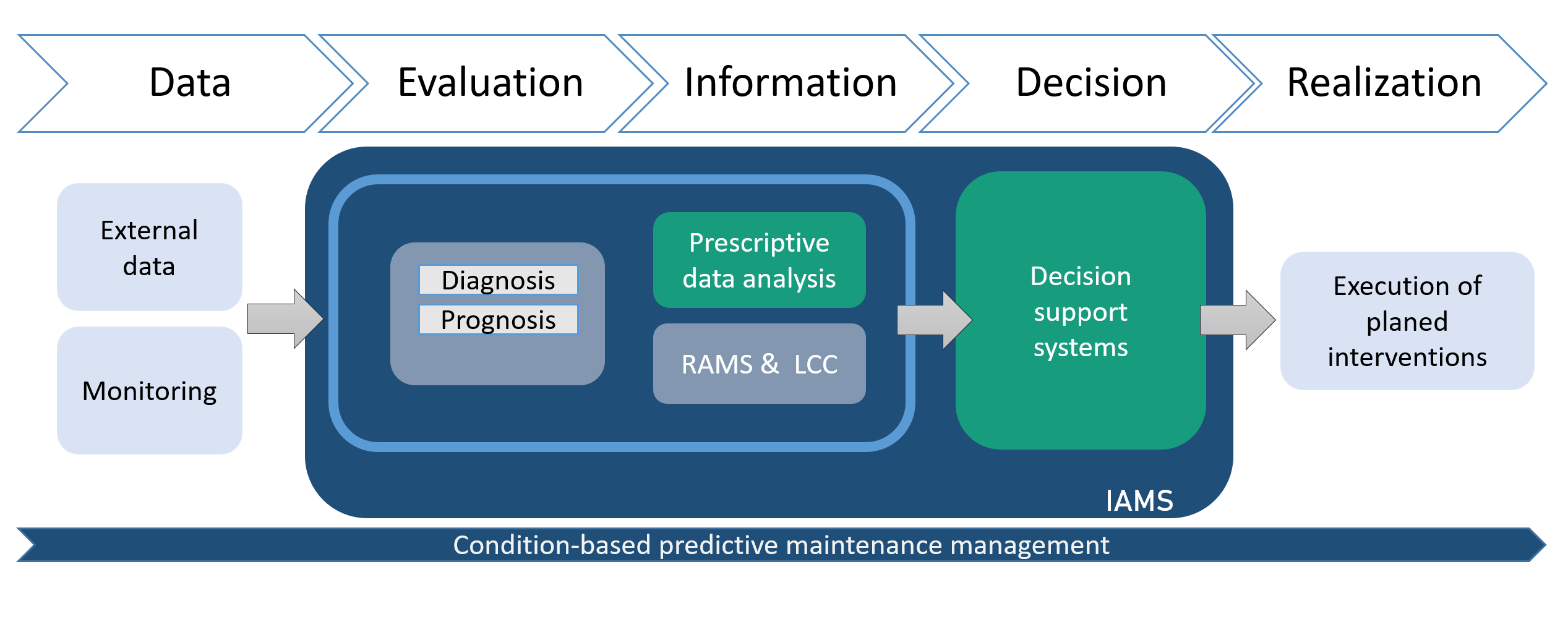
The IAMS (Intelligent Asset Management System) is a maintenance management system for railroad infrastructure systems. However, the methodology and systematics can also be applied to other maintenance problems. The aim is to facilitate and improve the decision-making process. IAMS supports the entire process chain from data acquisition to decision making. The starting point is measurement data resulting from the continuous recording of asset parameters or inspections. In addition, external data sources such as weather parameters or predicted asset utilization can also be taken into account. These data form the input for the IAMS.
The first step of the IAMS involves the evaluation of the measurement data, i.e., the diagnosis of the current state and the prediction of the future development.
There are two basic types of data evaluation: data-driven or based on expert knowledge. In the first variant, the historical and current measured values are used to evaluate the asset condition and its prediction. Generalized rules for diagnosis and prediction are derived from these correlations. In the second type of data analysis, these rules are based on expert knowledge. Both types have advantages and disadvantages, so the decision on how to analyze the data depends on both the availability and quality of the historical data and the expert knowledge available. In general, a combination of both methods provides the best results.
The generation of information necessary for planning is based on knowledge about the current and future condition of system components. Prescriptive data analysis examines which interventions are necessary to keep the system in a desired condition and which costs and risks are to be expected. In particular, life cycle cost (LCC) and reliability, availability, maintainability and safety (RAMS) analysis come into play.
This information forms the basis for various planning decisions at the three levels of maintenance planning: In the long term, maintenance and renewal strategies are defined in detail. In the medium term, the focus is on planning cost-intensive interventions that are characterized by long lead times. In the short term, detailed maintenance planning is carried out taking into account various framework conditions such as budgets, available maintenance teams, safety requirements or schedules/production plans, among others.
In the past, the Fraunhofer IVI has already developed various concepts and tools to support and automate decision-making processes in rail and road maintenance. These tools cover the following tasks: